Portfolio Model
The objective of the portfolio models is to build portfolios that are made available to investors and advisors in Deep Alpha Advisory Platform (DAAP). The portfolios are matched with the risk-levels. The portfolio models are consist of a set of asset classes/categories and forms the strategic asset allocation. There are two main ways of construting the portfolio model in DAAP:
- Manual portfolio model
- Optimized portfolio model
For FI that has defined weights and static model portfolios, the manual portfolio model can be a good fit. In these cases you will define the available asset classes and allocate weights, based on your investment philosophy. This can be illustrated in the example below, where we have three portfolios and 5 asset classes:
Main Asset Class | Sub Asset Class | Portfolio 1 | Portfolio 2 | Portfolio 3 |
---|---|---|---|---|
Fixed Income | Corporate bonds | 50 % | 25 % | ๏ปฟ |
Fixed Income | High yield bonds | 50 % | 25 % | ๏ปฟ |
Equity | Norwegian equity | ๏ปฟ | 10 % | 30 % |
Equity | Global equity | ๏ปฟ | 35 % | 60 % |
Equity | Emerging markets | ๏ปฟ | 5 % | 10 % |
You can build as many asset classes you want, and DAAP will suggest the correct portfolio, based on the riskmodel. If you have advisors using the platform, you can control the flexibility they have to deviate from these portfolios.
As an alternative to "Manual portfolio model", we offer the possibility to use our portfolio optimizer to construct the portfolio model. This will allow you for having a more dynamic set of portfolios, that will vary based on the market movements. The objective of the portfolio construction is to construct a portfolio, consisting of multiple asset classes, where the weights in the portfolio (portion of each asset) will be optimized to maximize expected return for a given risk (expressed as volatility).
Our portfolio optimizer use the modern portfolio theory (MPT), also known as Makowitz to opmize portfolios. MPT assumes that investors are risk averse, meaning that given two portfolio that offer the same expected return, investors will prefer the less risky one. Thus, an investor will take on increased risk only if compensated by higher expected returns. Conversely, an investor who wants higher expected returns must accept more risk.
There are some known limitations to MPT and we have implemented some measures (Constrains) to cope with them.
- Symmetrical risk. MPT threat a single big loss identical to many small losses while many investors will tend to avoid big losses. This can to some degree be mitigated by selecting instruments that avoid large downside deviation.
- Lack of diversification. MPT tend to put many eggs in the same basket. Normally constrains are needed to force the portfolios to contain more asset classes. However, this might affect expected return of the portfolio
- As for other models that use historical data, one can always discuss whether historical data is a good proxy for future performance or not. We allow for setting a custom lookback period, to be able to capture time periods that are relevant for the financial institution
A weight optimization accoring to MPT is on the bring of being unstable (chaos theory). The optimum is weak, and that results in two related issues:
- Many portfolios can provide almost identical results according to expected risk and return
- A minor change in performance in one or more benchmarks can alter the optimal weights significantly
We use constrains for multiple purposes:
- To avoid the recommended portfolio to change too much within short time frames
- Ensure enough diversity and avoid going all-in in one or few categories
- Limit exposure to equity
We have the following requirements for constrains:
- The sum of upper bound must be greater than 1
- Thre sum of lower bound must be lower than 1
- Sum of upper bounds in equity categories must be greater than MaxEquity
- Sum of lower bounds in equity categories must be lower than MaxEquity
- Sum of upper bounds in non-equity categories must be greater than 1-MaxEquity
- Sum of lower bounds in non-equity categories must be lower than 1-MaxEquity
- The categories must have risk (and correlations) that can span values of MaxRisk
MaxEquity = Maximum equity share for each risk class/portfolio MaxRisk = Maximum risk (volatility) for each risk class/portfolio
In order to optimize the portfolio, we are estimating the covariance matrix across all the categories included in the portfolio optimization. For estimation of the covariance matrix, we use a 20 year lookback period.
In DAAP we offer the possibility to include optionals as part of the portfolio optimization process. If optionals are included, we will reallocate parts of the equity fraction of the portfolio to the other asset classes. You can include up to 2 optionals at the same time:
- If 1 optional is selected, we will allocate 5 % to that particular asset class, and down-adjust the equity asset classes with the same fraction, and maintain the overall risk target
- If 2 optionals are selected, we will allocate 10 % to the two asset classes, and down-adjust the equity asset classes with the same fraction, and maintain the overall risk target
Optionals are often used to tilt the portfolio towards certain investor preferences. When you have included optionals in the portfolio optimization, you will get the following sets of portfolio out of the optimizer:
- Optimized portfolios without optionals
- Optimized portfolios with 1 optional
- Optimized portfolios with 2 optionals
For both portfolio modes, we are producing reports that you can use to get insight into the portfolios that are generated. We deliver the following reports:
- Risk matrix report - detaljert info om portefรธljene
- Category report - detaljert rapport per category
- Universe report - detaljert info om hver category + universe
In addition to these report, we produce a report that gives you insight into the instrument selection. More information about this can be found here: Instrument selection๏ปฟ Instrument Selection๏ปฟ๏ปฟ
The risk matrix report contains detailed information about the portfolios generated through the different modes. In addition to this, you will find detailed information about the covariance matrix used for estimating the historical portfolio risk and optimizing.
On category level you will get information about the following:
- Historical return
- Historical risk
- Weight in each of the portfolios
On portfolio level you will get information about the following:
- Estimated return (using historical return figures)
- Estimated risk (using covariance matrix and historical movements)
- Risk constrain
- Max equity constrain
- Equity portion
In addition to this, you will get detailed information about the unconstrained portfolio, that you can compare with your portfolio.
In addition to this, you will get a frontier plot, that illustrate the relationship between risk and expected return of the portfolio.
Example of a frontier graph is shown below:
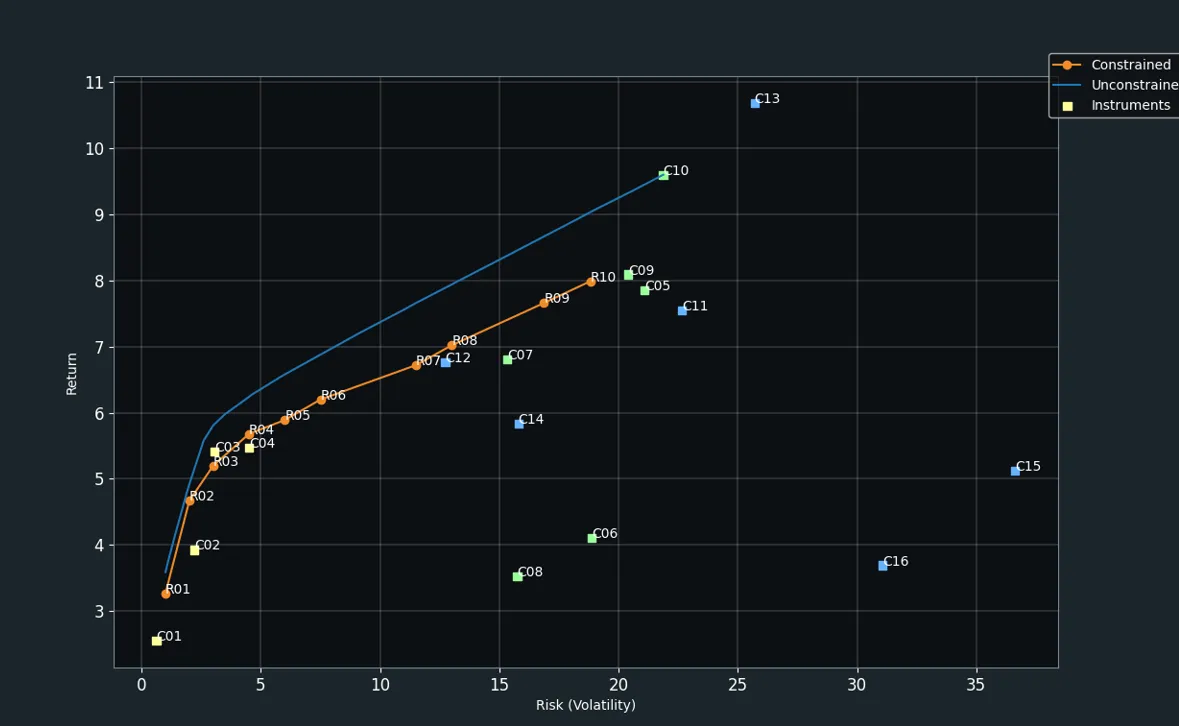
The category report gives you as a user detailed information about each of the categories you have included in the portfolio. For each of the categories included in the portfolios, this report will give you information about:
- Category ticker - ticker for the benchmark used for calculating the covariance matrix and building the portfolios
- Category ticker name
- Whether you are using a hedging the category or not
- Information about category used for patching the timeseries, if there is too little history available
- Detailed information about performance (risk and return) of the category and the belonging instruments
The universe report gives you basic information about the instruments that are available in each category.